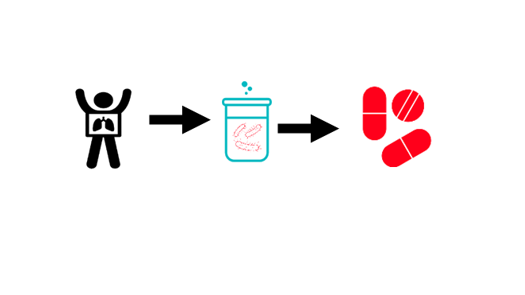
Computer-aided detection on chest X-ray for tuberculosis has high accuracy, does not depend on reported symptoms, has high throughput, and can thus be used to expand TB screening and diagnosis in high TB burden settings with limited chest X-ray expert readers
Why is it important to address the tuberculosis (TB) burden?
Tuberculosis (TB) is the second leading infectious cause of death globally after COVID-19. In 2020, 10 million people fell ill with TB globally and 1.4 million died of this disease. The global TB burden is gendered: men accounted for more than two thirds of TB cases in 2020. Diagnosis is the entry point into treatment and care for people with TB, however, an estimated 4.2 of the 10 million people who developed TB disease in 2020 were not diagnosed or reported, most of them men. This is an unacceptably high number and has likely increased due to the impact of COVID-19 and especially so in high TB burden countries.
Why is early diagnosis crucial to ending TB?
Early diagnosis and prompt treatment of people with TB is critical to the success of global efforts to end this awful disease by 2030. This is clearly stated in the World Health Organization’s End TB Strategy. Waiting though for people to recognise that they are ill and seek testing at health facilities misses many people with TB.
Strikingly, up to 50% of people, mostly men, found to have TB within communities deny having any TB symptoms. Men, compared to women and children, are likely to have TB without being aware of or reporting TB symptoms and have longer delays before seeking diagnosis. These delays can arise for multiple reasons, including individual and societal beliefs associated with being a man. In many societies, men are perceived as bread winners and may prioritise providing for their families, sometimes at the expense of health care seeking. Additionally, men who are perceived to be strong, may fear being perceived as weak if they seek health care for TB symptoms. Untreated people with undetected TB, who are likely to be men, continue to infect many other people, including women and children, within their communities.
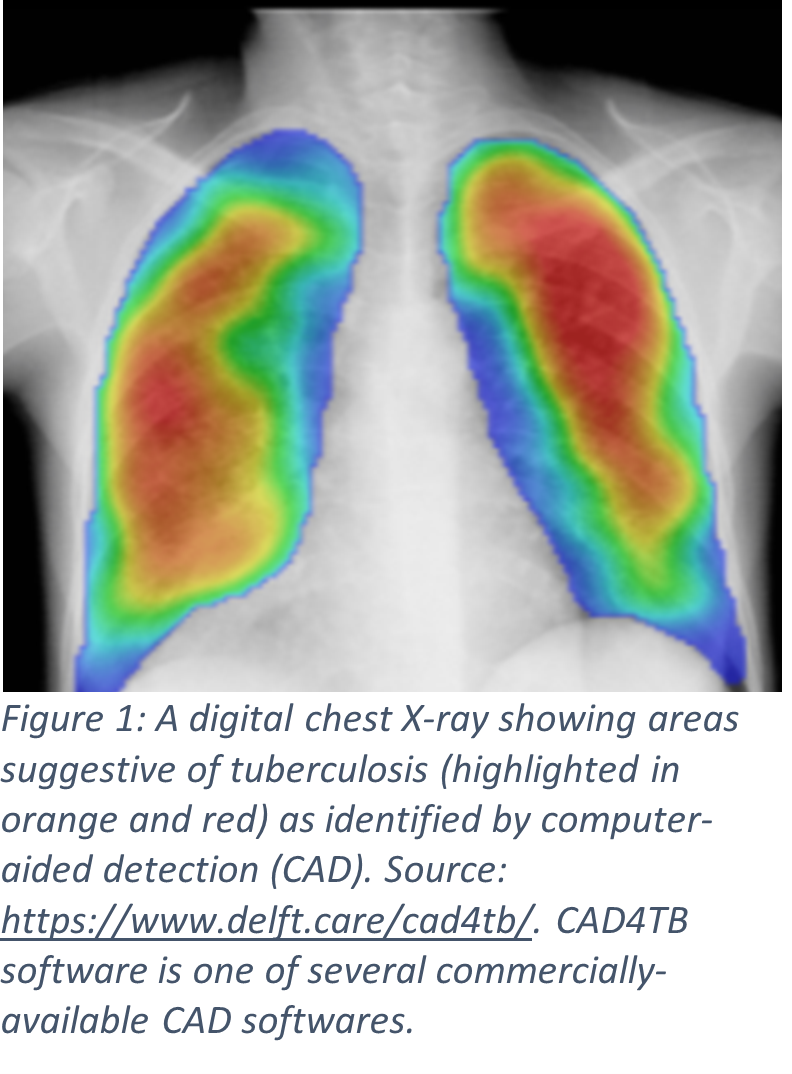
How can chest X-rays help to detect TB?
Excitingly, new approaches for expanding accurate TB diagnosis in the community may be here. TB primarily affects the lungs, causing damage which can be picked up on chest X-ray images. Computer-aided detection (CAD) on chest X-rays software can “read” X-rays of the lungs and output a score that indicates the likelihood of underlying TB (see Figure 1). People with scores suggestive of TB are then asked to provide sputum (or “spit”) to confirm presence of TB-causing bacteria. Those with confirmed TB are then offered treatment. So CAD on chest X-rays offered to people within communities and/or health facilities can identify people likely to have TB, who would otherwise be missed due to poor healthcare seeking behaviours, lack of symptoms or lack of screening and diagnostic resources (see Figure 2).
“Computer-aided detection does not depend on reported symptoms, has high accuracy and high throughput compared to human chest X-ray readers.”
Dr Peter MacPherson, TB expert and researcher, Malawi-Liverpool-Wellcome Trust Clinical Research Programme and LSTM
CAD is as accurate as expert human readers, does not rely on reported symptoms and is fast giving a result within 1 minute. This is particularly important in countries where TB is common, as often there are very few doctors or X-ray specialists available to interpret scans. In Malawi, southern Africa, and several other countries, CAD has been rigorously tested in trials. CAD can be used without specialist expertise as part of mobile TB screening interventions and thus achieve high throughput compared to human chest X-ray readers. Since 2021, the World Health Organization recommends the use of CAD for TB screening and triage with several CAD software options available for use by national and global TB programmes. In a very engaging Connecting Citizens to Science podcast, Dr Jacob Creswell from the Stop TB Partnership and Dr Brenda Mungai from the Liverpool School of Tropical Medicine provide insights into how CAD has revolutionised TB screening and diagnosis.
What are the global targets for TB case detection and how could CAD help to reach these targets?
TB screening aims to maximise the number of TB cases detected and linked to treatment and care. By 2035, the WHO End TB Strategy aims to reduce the number of new TB cases by 90% compared to 2015 levels of less than 10 per 100,000 population. CAD offers renewed hope for achieving this aim by expanding entry points for diagnosis, treatment, and care, for people who would otherwise not be detected and reduce onward transmission.
What is the potential population-level impact of using CAD for TB screening on TB transmission, morbidity, and mortality?
As part of the LIGHT Consortium, researchers at Malawi-Liverpool-Wellcome Trust Clinical Research Programme will use modelling to estimate the number of infections, sickness episodes and deaths that could be prevented by using CAD-based screening strategies compared to non-CAD screening approaches. Mathematical “models” are a helpful tool for mimicking reality and compare possible scenarios where various infectious disease control interventions are implemented, and thus evaluate their impact. Mathematical models are useful for understanding the wider population-level impact of interventions, implemented alone or in combination, otherwise elusive to measure in field studies. For example, a TB screening intervention implemented at a single time point, in a high TB burden setting, not only reduces current TB disease burden, but also consequently reduces future instances of TB transmission and disease, beyond people initially targeted by the screening intervention. Measuring the immediate and future impact of such screening interventions, though possible in a field survey, requires a sufficient time lag for the effect of the screening to be realised, can be costly, and can be challenging to disentangle in the context of contemporaneous control interventions. Mathematical models can mitigate against these challenges and provide timely evidence to inform decisions made by national and global TB control programmes.
What are the potential implications of findings from our study?
Findings from this research will help to inform decision-making for using CAD-based strategies as an efficient means to diagnosing and treating otherwise undetected TB cases, and thus reduce transmission and mortality where the burden of TB is high. Whilst there is an initial focus on Malawi and Kenya, two African countries with high TB burden, these approaches and insights may be useful for other national programs considering widescale use of CAD for TB screening.
Authors: Mphatso Phiri, Peter MacPherson, Marc Henrion and Katherine Horton
This is the first blog in a series that examines gender-sensitive TB interventions in Malawi, drawing on the research of The LIGHT Consortium.